Coauthored by Ben Xue and Yi Zhang
This is the third entry in a series of articles focused on AI/ML.
Natural language processing (NLP) is a form of artificial intelligence (AI) that gives machines the ability to read, understand, and derive meaning from human languages. NLP powers many applications that we use every day, such as virtual assistants, machine translation, chatbots, and email auto-complete. The technology is still evolving very quickly. Just over the last few years, we have seen incredible breakthroughs in NLP research, including transformers and powerful pre-trained language models such as GPT-3, which have significantly accelerated the development of NLP applications in various domains.
At Netskope, we are integrating the latest NLP technology into our secure access service edge (SASE) solution, as well as business operations. NLP is behind the scenes for a wide variety of tasks, including:
- Detecting sensitive information in documents to help our customers comply with privacy regulations and protect their digital assets.
- Categorizing and detecting malicious web domains, URLs, and web content to enable web filtering.
- Detecting malware and protecting enterprise assets from being compromised and used as a launchpad for malicious activities.
- Classifying SaaS and web apps and evaluating the enterprise readiness of a cloud app as part of the Cloud Confidence Index (CCI).
In this blog post, we will highlight three ways Netskope uses NLP to secure data and protect against threats: DLP document classification, URL categorization, and DGA domain detection.
DLP Document Classification
Various documents from our customers are stored in their cloud storage or transferred through cloud applications. Many of these documents contain sensitive information, including confidential legal and financial documents, intellectual property, and employee or user personally identifiable information (PII). At Netskope, we have developed machine learning-based document classifiers, as part of our inline Data Loss Prevention (DLP) service. The ML classifiers automatically classify documents into different categories, including tax forms, patents, source code, etc. Security administrators can then create DLP policies based on these categories. The ML classifiers work as a complementary approach to traditional regular expression-based DLP rules and enable granular policy controls in real-time. In many cases, manually configured regex rules can generate excessive false positives or false negatives when looking for specific patterns in documents. In comparison, the ML classifiers automatically learn the patterns and identify sensitive data in real-time, without the need for traditional DLP rules.

Text classification is one of the standard NLP tasks. As illustrated in Figure 1, we extract the text content from documents and use a pre-trained language model as an encoder to convert documents into numeric values. Based on the document encodings, we then train document classifiers in the form of fully connected neural network layers. Currently, the classifiers are able to accurately identify more than 10 types of documents with sensitive information, including:
- Source code
- IRS tax forms
- M&A forms
- Resumes
- US patent files
- Offer letters
- Bank statements
- Non-disclosure agreements
- Consulting agreements
- Partner agreements
- Stock agreements
- Medical power of attorney forms
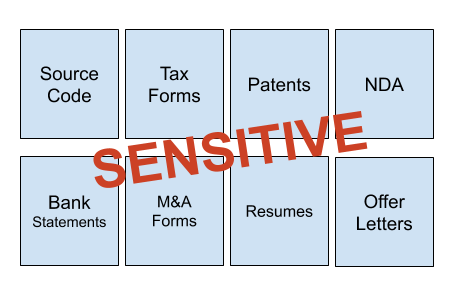
The light-weighted document classifiers are able to run inline to provide real-time data protection for our customers.
URL Categorization
Web content filtering helps organizations to regulate access to websites that may have offensive, inappropriate, or even dangerous content. NLP-based URL categorization is responsible for grouping websites into different categories based on their text content, which enables web content filtering.
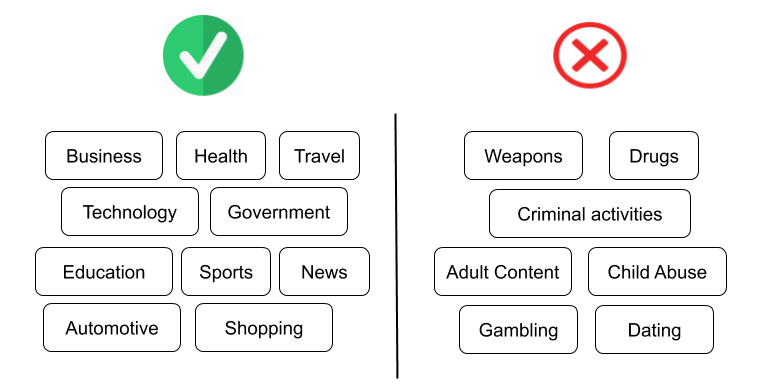
Traditionally, a text classification machine learning model is trained for a specific language. With the latest development in NLP, it is possible to train a multilingual classifier that supports multiple languages. The training data can be a mixture of text in different languages, and the trained model can predict the category of the new text, regardless of which language it is expressed in. We have developed multilingual URL classifiers with the state-of-the-art transformer language model BERT that supports over 100 languages. Based on the content that is crawled dynamically, the classifiers accurately identify websites in many undesirable categories, including weapons, drugs, adult content, criminal activities, etc.
DGA Domain Detection
Modern malware, such as botnets, ransomware, and advanced persistent threats, typically makes use of a domain generation algorithm (DGA) to avoid command and control domains or IPs being seized or sinkholed. It is important to detect DGA domains automatically in order to block malicious domains and identify compromised hosts. Traditional DGA detection techniques rely on collecting the contextual information (e.g., IP, NXDomains, HTTP headers) of the domains and blacklisting. In comparison, machine learning-based DGA domain detection has the potential to identify unknown DGA domains.
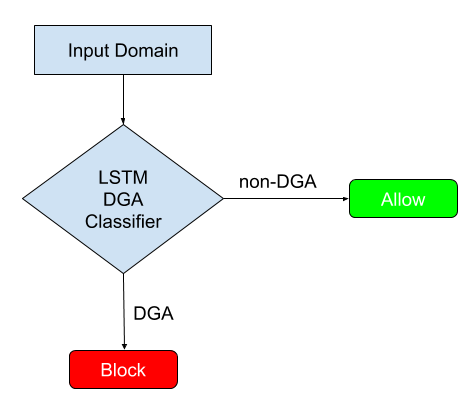
On the surface, determining whether a domain such as intgmxdeadnxuyla.com is DGA or not has nothing to do with natural language processing. Actually, it is very similar to an NLP task if we treat each character in the domain name as a word and the full domain as a sentence. We can then use NLP techniques to learn the semantic relationship between the characters and the overall meaningfulness of the domain. We have developed a DGA domain classifier based on Long Short-Term Memory Networks (LSTM), a Recurrent Neural Networks architecture commonly used in NLP. Based on millions of training samples, the LSTM classifier captures the context information in each domain by treating it as a sequence of characters and classifies it as DGA or non-DGA with high accuracy.
Future of NLP
This is a golden era for natural language processing. NLP models are getting faster and more powerful by the day. At Netskope, we will provide better data and threat protection to our customers with the latest NLP technology. What problem are you trying to solve? Contact us at [email protected] to share it with us.